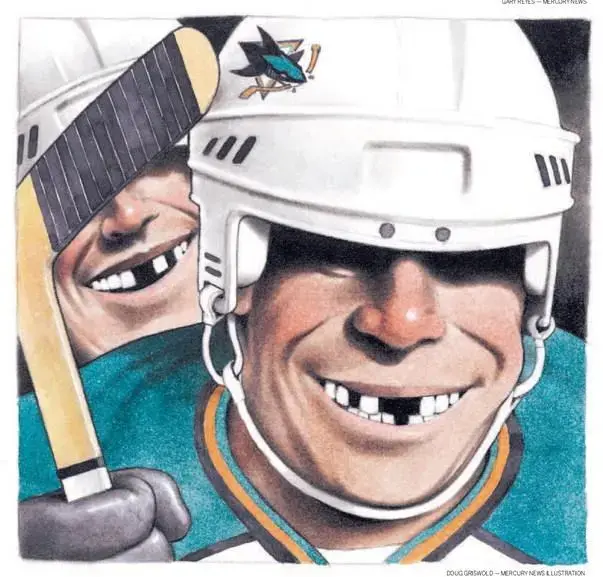
The marketing director approached me after I had presented our work in multi-channel ad effect measurement at a large telco. I had not met him before and he worked for another business unit to the one that had hired us.
He was almost embarrassed to ask the questions that were clearly bothering him. We sat down over coffee and he told me his story.
He was responsible for direct and digital marketing to the telco’s existing customers, selling a service to add to the one(s) they already had. His channels were email and addressed direct mail. Since his budget was limited, he asked the in-house modelling group to help him target his campaign, asking who should get both an email and a DM? What he showed me was a report of new service activation rates by model decile, after the last campaign had finished.
Before we consider what he and I were looking at, let’s start with what a good targeting model should deliver in a case like this. Such a model should use data about customers: description and their history of purchases, cancellations, billing and customer service interactions. It should use the communication history for each individual customer; what channel was used to contact him/her, when, for what product, what offer and what messaging and any interactions that had resulted from campaigns used in the past. In the case shown below, this particular model also uses neighbourhood demographics.
Consider the following post-campaign report, from a telco client of ours, also for a customer cross-sell campaign. Predicted scores are the probability of activation (the customer orders and installs the product) for the campaign period (here, 4 weeks after first contact). These scores were generated about 12 weeks before the campaign was launched; enough time to prepare the mailers. Actual scores are recorded for the 4 week campaign window, against both non-communicated and non-targeted control groups (our take on control group design for CRM will be covered in a future blog post).
There are three features of this report we should pay attention to: (deciles represent scores for each customer, averaged)
1. Each decile shows predicted scores that are lower than the decile before; think of this as a “ski slope” shape.
2. There is excellent differentiation between predicted scores for the best decile vs the average (here, 3.1 times) or vs the lowest decile (here, 15.3 times). The more differentiation, the more opportunity we have to create business value.
3. There is a close correspondence between predicted and actual by decile. The model does not over- or under-predict for any given decile. This level of accuracy allows us to invest with confidence.
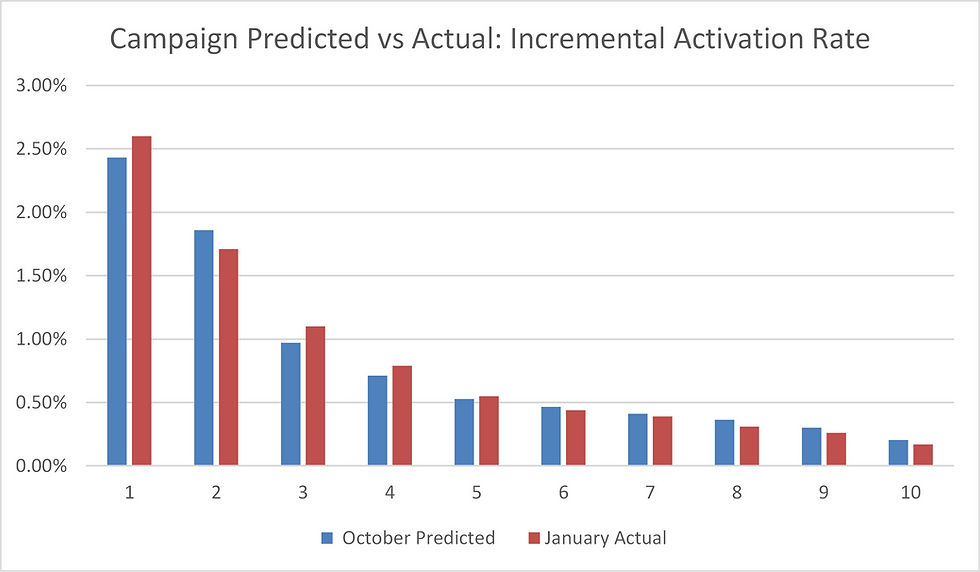
To the predictions we can now add the cost of contact (here, DM + EM = $3.00) and value of activation (here, $75.00), which gives this picture, for a base of 5 million customers (outcomes assume we contact all customers in each decile):
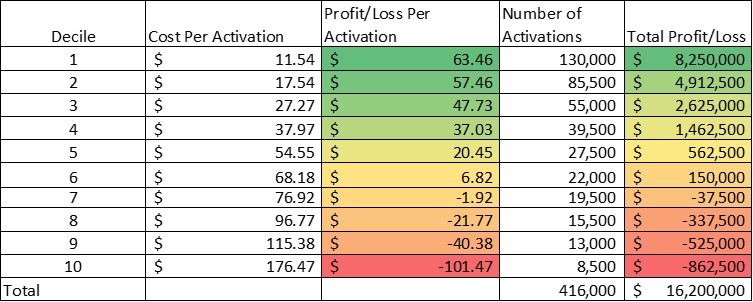
For planning purposes, we would select only the top 7 deciles in order to maximize profit.
(For a future blog post: how we can use this as a starting point to create even more value)
Now, let’s consider the picture our director of marketing was looking at. I mocked up the report, here, from memory.
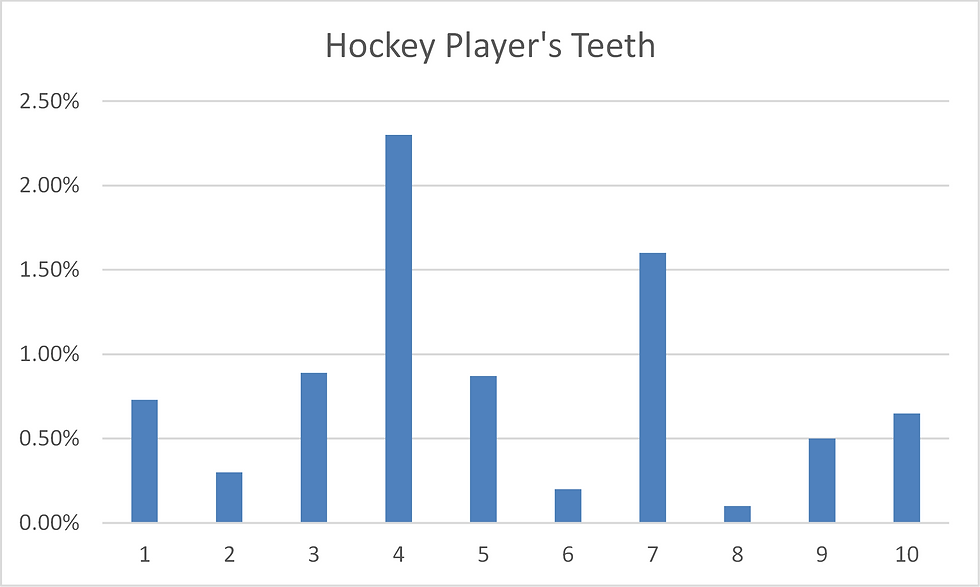
As you can see, the best decile for activation rate is NOT the first...it is the fourth. The first, which should be the best, is the 6th worst. The 7th has good performance...who would have thought? In short, instead of seeing the “ski slope” shape we see with a good model, this report looks like a hockey player’s teeth after the playoffs.
You cannot make good targeting decisions using this model. Why was it performing this way?
I went next to see a senior member of the modelling team and we quickly agreed on the cause: the model had no communication variables at all. Instead of being able to ask the question, “what would be the predicted activation rate if I contacted a given customer, with a given channel”?, the model the marketing director was given provided scores that had no relationship to the outcomes he wanted.
Why was it designed this way? Because the modelling group felt that their scores reflected a prioritization of customers for whom a sale of this type would be most likely to improve retention and generate long term cash flows. While this is useful as a way to study customer behaviour, it is useless when it comes to designing a marcom campaign, which was their brief. The modelling group felt that the director of marketing had to “learn” how to sell to the top deciles. How? That question went unanswered.
I often say in the analytics business there is a substantial knowledge gap between the people building models and the decision-makers using them. This gap should be closed with clear, transparent communication. In this case, that didn’t happen; the modelling group pushed a solution that fit their preferences, even knowing it was not what was asked for.
In cases like these, such models are more likely to get their marcom users fired than promoted. And that is a failure for all involved.
DB
Comments