Will that model get you promoted? Or fired? (Part 1)
- dbeaton9
- Oct 7, 2024
- 4 min read
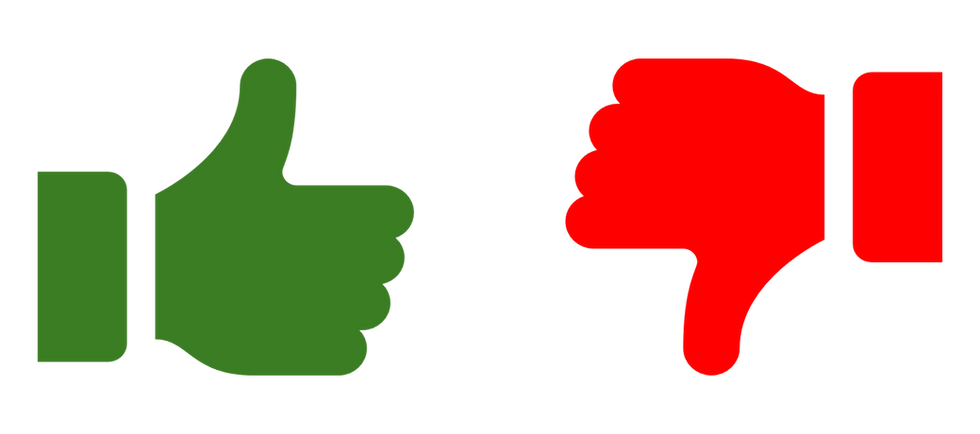
Robert Jones, like many of us, trusted his car’s sat-nav system to help guide him to his destination. But on one unfortunate day, it almost cost him his life.
On that day, Mr Jones followed sat-nav instructions so closely he dismissed the evidence of his own eyes. The narrow, steep path he was driving on was clearly unsuitable for a car, but the system told him it was a road. He almost plunged his car off a 100 ft cliff as a result[1].
That same year, the US National Highway Safety Administration estimated that GPS systems caused over 200,000 accidents every year in the United States[2].
Can we really trust the data and models that we use to navigate our lives? How do we know when we can, and cannot?
Marketers live in a complex world, and seek to create change in that world. In doing so, they grapple with problems of targeting, segmentation, evaluation, optimization and diagnostics. Increasingly, they turn to sophisticated analytical technology to disentangle data in search of reliable cause and effect quantification.
Are those models leading marketers to good outcomes, reliably, consistently, or leading them off the proverbial cliff?
There is an imbalance of knowledge between those building advanced analytics systems and their marketing decision-maker users. The former understands the math and the data, but may not fully grasp the risks. The latter assumes the risks of using models without, in many cases, having the quantitative skills to assess those risks themselves. Some don’t even know they are taking on risk, or how much. How can marketers and advertisers protect themselves from the downsides some forms of advanced analytics expose them to?
One should not need to be a Ph.D. in math or stats to use an MMM or CRM model as a client. But one should be able to do a basic check of the work, enough to spot weaknesses and avoidable risks.
There are three I recommend to my clients:
· Data: what data is used to build the model
· Accuracy: how accurate is the model
· Actionability: how easily and effectively can the model’s prescriptive analytics be implemented?
Let’s do a high level review of each, in the context of a Marketing Mix Model use case.
Data: what data is used to build the model
Before building an MMM, you should satisfy yourself that the right data goes into its construction, not just the easy data, that is, data easily available. Ask yourself what factors causes changes in the outcomes you are interested in. For an MMM, these would certainly include paid media, but you should also include owned and earned media as well. And you should include data on factors that may be out of a marketer’s control but that affect outcomes strongly such as weather or local economic conditions. Take a holistic approach to understanding what drives outcomes. Not all factors tested in the model may be significant, but taken together they are more likely to produce a model you can trust than if major drivers are ignored.
Today, many advertisers rely on digital attribution systems that break this rule by focusing on digital media data only. Those results should be treated with great caution.
Accuracy: how accurate is the model
If a model can reproduce the time series outcome data on your business with a high degree of accuracy, we believe it is fairly quantifying those cause-and-effect relationships that shape those outcomes. But how accurate does it need to be?
Consider this case. This brand does $1.2 billion in sales per year; you see three years here in time series. As you can see, the predicted sales numbers, from our model, almost matches the actual. We explain 95% of the variation in sales we see over this time....not just the broad trend of the business but the monthly nuance.
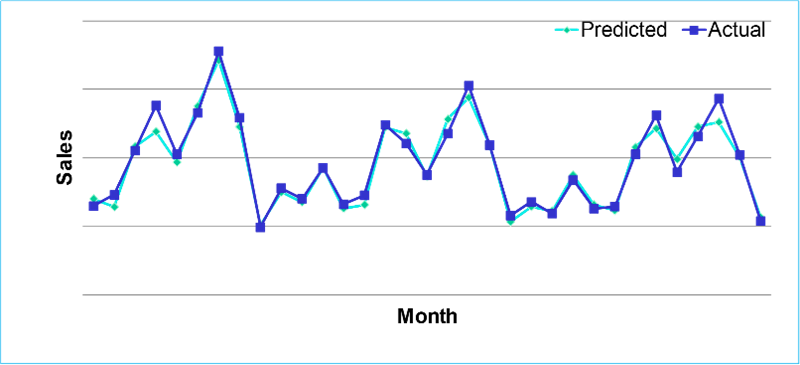
In our work, we advocate accuracy rates at or over 90% over the calibration period, validated by an out-of-period test of fit.
The higher the accuracy, the lower the risk of mis-attribution and of making bad forward-planning decisions. Cause-and-effect are properly quantified and effects dis-entangled. Marketing and advertising decisions can be made with greater confidence and good outcomes follow.
Actionability: how easily and effectively can the model’s prescriptive analytics be implemented?
Consider how easy, or how difficult, it is to make changes to your marcom decisions using the model. The more directly the model reflects how media is actually planned and bought, the easier it will be to implement. When a model is overly theoretical, it is too hard to buy according to its recommendations and opportunity is missed.
Implementing an inaccurate model, built on incomplete or unreliable data, and having a poor alignment with actual buying practice can leave you worse off than having no model at all (future blog post!).
Carefully test your model on all these questions, and you will find you are more likely to reach your objectives, instead of finding yourself teetering on the proverbial cliff.
Footnotes:
[2] Science & Justice Journal, Vol 63, Issue 3, May 2023, Pages 421-426
Kommentarer